A critical eye on technology.
In-depth analysis, open-source tutorials, and high-tech news to help you understand the digital world, not just endure it
Read our articles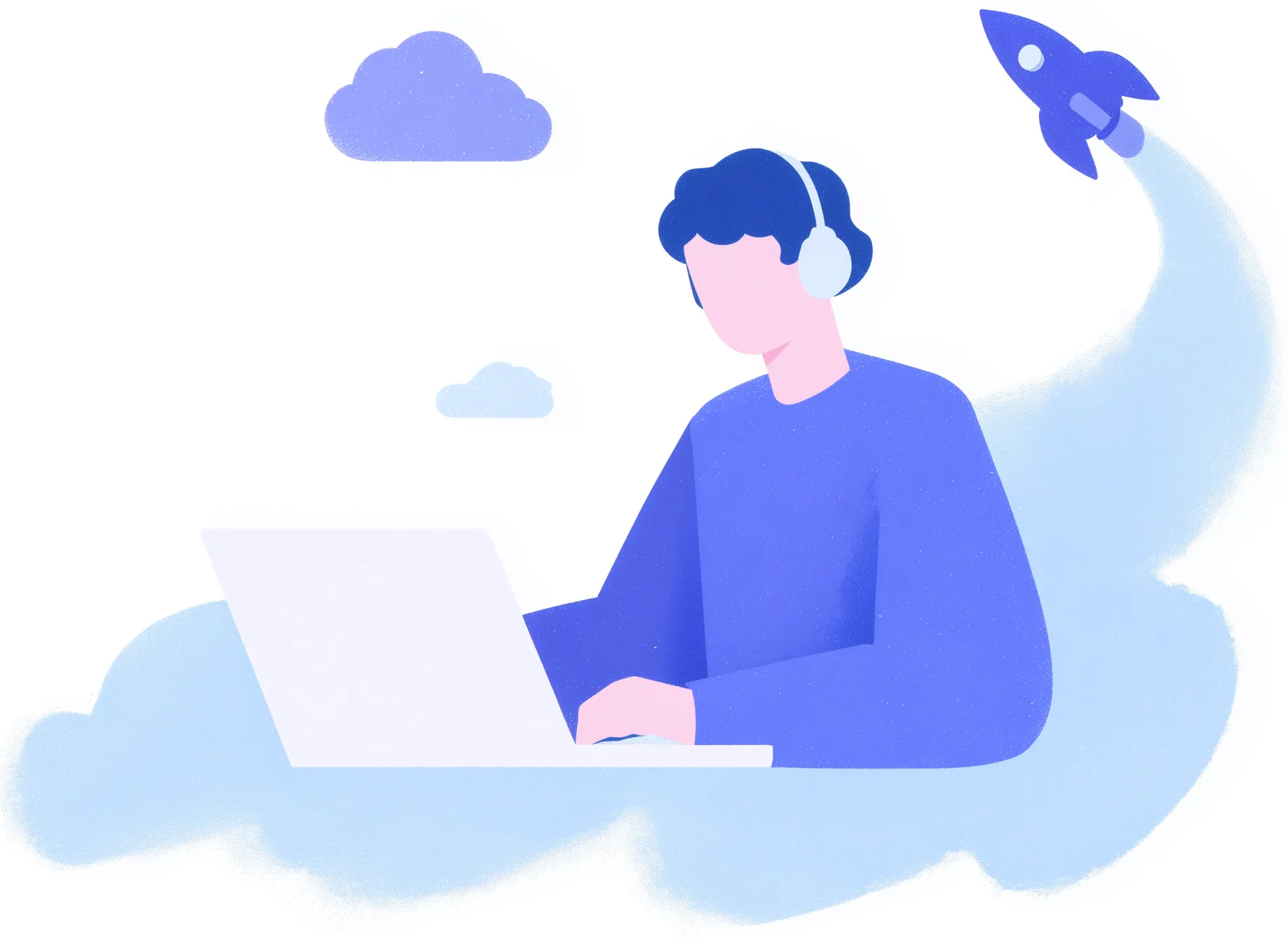
LATEST ARTICLES
Beyond the Hype
In a world saturated with marketing and technical complexity, telling the signal from the noise has never been more challenging. At Cynic.cc, our mission is simple: to provide you with the critical insight and technical expertise needed to see beyond the surface.
Our in-depth analyses break down the strategies of tech giants, our open-source tutorials empower you with practical skills, and our coverage of AI news helps you navigate the ever-evolving digital world.